Defense against the Darknet, or how to accessorize to defeat video surveillance
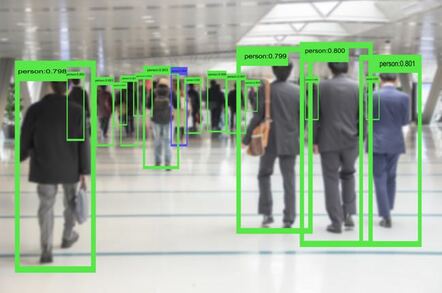
In a research paper distributed through ArXiv in advance of its presentation at computer vision workshop CV-COPS 2019, Simen Thys, Wiebe Van Ranst and Toon Goedeme from KU Leuven describe how some colorful abstract signage can defend against Darknet, an open source neural network framework that supports You Only Look Once (YOLO) object detection.
The paper is titled "Fooling automated surveillance cameras: adversarial patches to attack person detection."
Adversarial images that dupe machine learning systems have been the subject of considerable research in recent years. While there have been many examples of specially crafted objects that trip up computer vision systems, like stickers that can render stop signs unrecognizable, the KU Leuven boffins contend no previous work has explored adversarial images that mask a class of things as diverse as people.
"The idea behind this work is to be able to circumvent security systems that use a person detector to generate an alarm when a person enters the view of a camera," explained Wiebe Van Ranst, a PhD researcher at KU Leuven, in an email to The Register. "Our idea is to generate an occlusion pattern that can be worn by a possible intruder to conceal the intruder from for the detector."
No comments:
Post a Comment